How AI is improving natural disaster predictions
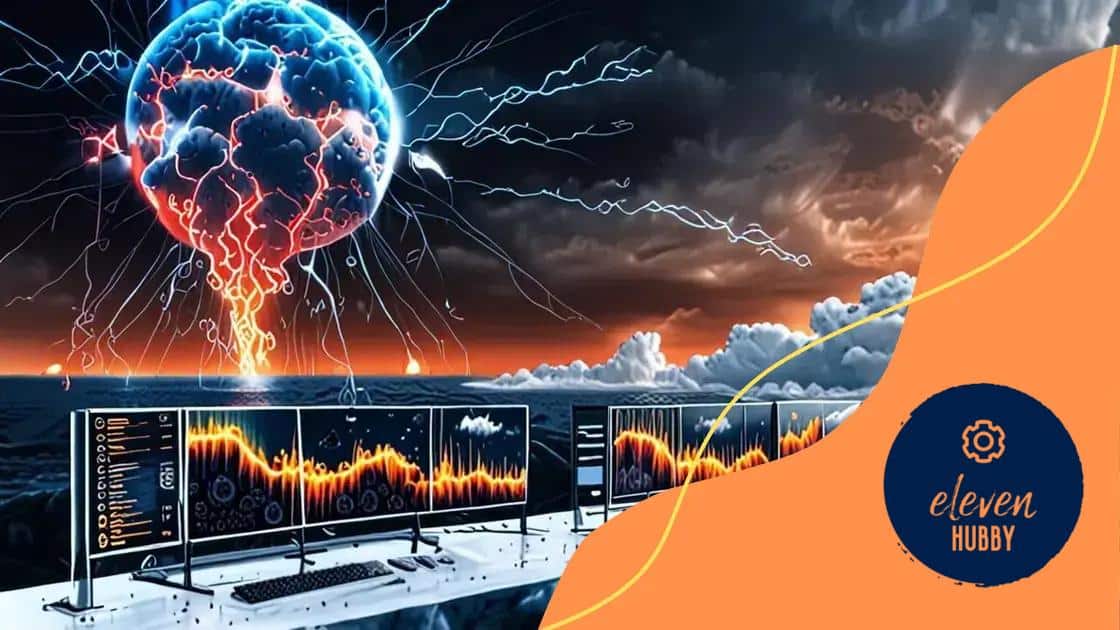
AI is improving natural disaster predictions by utilizing big data analytics, machine learning algorithms, and real-time data from IoT devices to enhance accuracy and responsiveness in emergency management.
How AI is improving natural disaster predictions is a topic that sparks curiosity as it merges technology with life-saving strategies. Have you ever wondered how we could anticipate calamities better?
Understanding AI in disaster predictions
Understanding how AI plays a role in disaster predictions is vital. The integration of artificial intelligence into forecasting systems has changed how we anticipate and respond to natural disasters.
The Basics of AI in Disaster Prediction
At its core, AI technology uses algorithms and data analysis to recognize patterns. By studying past disaster data, AI systems can offer forecasts that are more accurate than traditional methods.
These systems analyze multiple factors, including weather changes, geological data, and historical patterns. Such insights empower authorities to plan effective responses in advance.
Key Components of AI Disaster Systems
- Data Collection: Gathering vast amounts of data through sensors, satellites, and public records.
- Machine Learning: AI algorithms learn from data and improve their predictions over time.
- Real-Time Analysis: AI evaluates and predicts disasters in real-time, allowing for immediate alerts.
- Visualization Tools: These help convey critical information swiftly to decision-makers.
The effectiveness of this technology stems from its ability to adapt and learn from new data continuously. For example, when a new weather pattern emerges, AI systems can reassess and adjust predictions accordingly, ensuring they remain accurate.
Moreover, the implementation of AI in this field is already showing promising results. In several instances, communities have utilized AI-driven predictions to enhance their readiness, saving lives and minimizing property damage.
Overall, understanding AI in disaster predictions opens up exciting possibilities. As technology advances, we can expect even greater improvements, making our world safer and more prepared for unforeseen events.
The technology behind AI predictions
The technology behind AI predictions is fascinating and multi-layered. It combines various disciplines, including computer science, data analytics, and even cognitive science. Understanding these elements can help us grasp how AI effectively predicts natural disasters.
Data Collection and Integration
The first step in AI predictions involves data collection. AI systems gather data from diverse sources like satellites, ground sensors, and social media. This wealth of data is crucial for building accurate models.
Integrating these data sources helps create a more complete picture of environmental conditions. By merging information, AI can detect patterns that would be difficult to spot using traditional methods.
Machine Learning Algorithms
Once data is collected, machine learning algorithms come into play. These algorithms analyze the data to identify trends and make predictions. For example:
- Supervised Learning: This approach uses labeled datasets to teach the AI how to predict outcomes.
- Unsupervised Learning: Here, the AI finds hidden patterns without pre-existing labels.
- Reinforcement Learning: In this method, the AI learns from its mistakes and successes to improve future predictions.
Through these processes, AI becomes adept at forecasting natural disasters by recognizing subtle signs that might indicate an approaching storm or earthquake.
Additionally, the use of advanced algorithms allows for real-time processing. This means that as new data comes in, the AI can quickly update its predictions, providing timely information for emergency planners and responders.
Understanding the technology behind AI predictions reveals not only how it functions but also its potential to save lives. By leveraging these tools, we can prepare better for natural disasters and potentially mitigate their impacts.
Case studies of AI saving lives
Case studies of AI saving lives demonstrate the real-world impact of this technology. Numerous examples show how AI has transformed disaster response and preparedness.
Hurricane Predictions
In recent years, AI has significantly improved predictions for hurricanes. For example, Florida implemented AI-driven models to track storms more accurately. By analyzing large datasets, these models provide timely alerts, allowing residents to evacuate early.
In one case, AI predictions helped emergency services prepare for Hurricane Dorian in 2019. As a result, the state was able to allocate resources efficiently, saving countless lives.
Earthquake Detection
Another impressive application of AI is in earthquake detection. AI systems monitor seismic activity and can predict tremors within seconds. In Japan, for instance, AI algorithms analyze real-time data from multiple sources, improving warning time before an earthquake strikes.
This technology has proven vital; during a 2020 quake, residents received alerts just seconds before shaking began, giving them time to seek shelter.
Flood Forecasting
AI has also made strides in flood forecasting. Using machine learning, forecasters can analyze weather patterns to predict flash floods accurately. For instance, the National Oceanic and Atmospheric Administration (NOAA) utilized AI to enhance its flood models.
- Increased accuracy: The AI system improved prediction accuracy by 30%.
- Early warnings: Communities received advanced warnings, helping them prepare for potential evacuations.
- Resource allocation: Emergency services managed resources more effectively, preventing loss of life.
These case studies illustrate that AI is not just a theoretical concept; it has tangible effects on saving lives. By leveraging data and advanced technology, we can enhance our response to natural disasters.
Challenges in AI for natural disasters
Challenges in AI for natural disasters are significant and can impact the effectiveness of disaster response systems. While AI offers great potential, understanding its limitations is equally important.
Data Quality and Availability
One major challenge is the quality of available data. AI systems rely heavily on accurate and comprehensive data to make reliable predictions. However, in many regions, especially developing areas, data might be sparse or outdated. This lack of quality data can lead to incorrect forecasts.
For instance, if the data used for predicting flooding is not up-to-date, the AI might fail to identify recent changes in weather patterns, which are critical for accurate predictions.
Integration with Existing Systems
Another challenge lies in integrating AI technologies with existing disaster response systems. Many emergency services still use traditional methods for tracking and responding to disasters. Transitioning to AI-driven models requires considerable effort, training, and resources.
- Training personnel: It is essential to train responders on how to use AI tools effectively.
- System compatibility: Ensuring that AI systems work well with older technology presents technical hurdles.
- Resource allocation: Allocating necessary resources for integration can strain budgets.
Furthermore, reliance on technology can introduce vulnerabilities. In high-stress situations, technical failures might hinder communication and decision-making, potentially leading to dire consequences.
Ethical Considerations
Ethical concerns also play a critical role. For example, how decisions are made by AI systems can raise questions about accountability. If an AI system provides a poor prediction leading to loss of life, who is responsible? These questions complicate the implementation of AI in disaster scenarios.
Moreover, there’s the issue of bias in data. If the data used to train AI models reflects historical prejudices, the resulting predictions may also be biased, putting certain populations at greater risk. Addressing these challenges is essential for ensuring equitable and effective disaster response.
Future trends in AI disaster management
Future trends in AI disaster management highlight the exciting developments on the horizon. As technology continues to advance, we can expect AI systems to become even more adept at predicting and responding to natural disasters.
Enhanced Predictive Analytics
One of the most promising trends is the improvement of predictive analytics. By combining AI with big data, disaster management systems will generate more accurate forecasts. Advanced algorithms can analyze weather patterns in real-time, leading to quicker and more precise warnings for communities.
Moreover, machine learning will continuously refine these predictions. As AI systems learn from past events, they will enhance their effectiveness, helping first responders prepare better.
Integration with IoT Devices
The rise of the Internet of Things (IoT) will revolutionize disaster management. Smart devices, like sensors and wearables, will collect vast amounts of data that can feed into AI systems. This data stream will enhance situational awareness during a disaster.
- Real-time monitoring: AI can analyze data from IoT devices for instant updates.
- Automated alerts: Smart devices will notify users of imminent dangers based on AI predictions.
- Resource tracking: AI will help locate and manage resources more efficiently during an emergency.
This integration will empower communities to respond more effectively and save lives during disasters.
Collaborative AI Systems
Another significant trend is the development of collaborative AI systems. These systems will share data across various regions and agencies, improving coordination during disaster responses. When different organizations can access the same information, they can work together more effectively.
AI will also facilitate simulations and training exercises, allowing agencies to practice responses in virtual environments. This preparation will strengthen real-life responses when disasters strike.
As we look to the future, AI disaster management will continue to evolve. The combined potential of enhanced analytics, IoT integration, and collaborative systems promises a safer world for all.
FAQ – Frequently Asked Questions about AI in Natural Disaster Management
How does AI improve disaster predictions?
AI improves disaster predictions by analyzing large datasets to identify patterns, leading to more accurate forecasts and timely alerts.
What role do IoT devices play in disaster management?
IoT devices collect real-time data that enhances situational awareness and helps AI systems make informed predictions during disasters.
What are some challenges of implementing AI in disaster response?
Challenges include data quality issues, integrating AI with existing systems, and addressing ethical considerations around accountability.
What future trends can we expect in AI disaster management?
Future trends include enhanced predictive analytics, greater integration with IoT devices, and collaborative systems for improved response coordination.